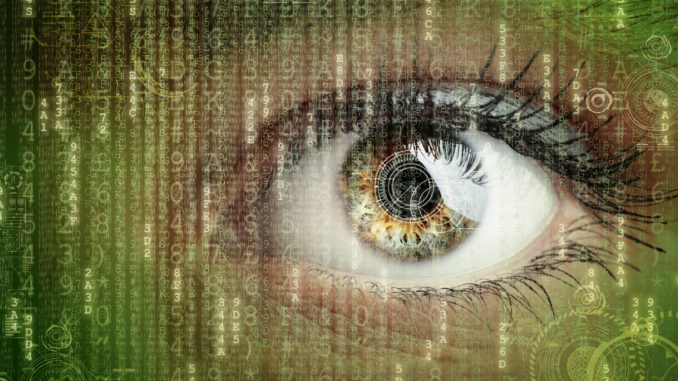
By Shammy Narayanan
A few days back, I took to LinkedIn to highlight the culinary catastrophe of mutton Biryani being crowned a “Non-Guilty” pleasure on a food delivery app. In a world where Biryani is practically an emotion♥️ , the post stirred quite the pot, and readers fell into two camps. Some called out the mislabeling, while others argued it’s all a matter of perspective. Kudos to the latter for their spirited defence—it was like watching Olympic-level mental gymnastics! The debate rages on.
But it got me thinking. If we can’t agree on the labelling/ classification of a singular data item within a small group, how on earth do we expect multiple teams and diversified stakeholders to see eye-to-eye in interpreting volumes of data? Aside from the usual suspects of null and missing values, is Data Quality (DQ) really a technical quagmire, or is it, dare I say, a people problem?
Snapshot Indicating Briyani as Guilt-Free Food
Data quality is not an absolute term. Let me substantiate it with an example of a claim dataset. Imagine the data as a shape-shifter! When the use case is hunting for popular Claim submission counties, having a valid zip code makes the dataset a hero – we call it “Good Data.” But when it’s on a mission to uncover non-primary symptoms and clinical codes, suddenly, these same claims go from Hero to Zero, needing a trio of details to stay in the game. The data remains unchanged, but its worth depends on what we’re looking for. The first task gives it a high-five, while the second gives it a timeout. So, Data quality is merely a function of the Usecase?
Time, my friend is also a player in this DQ game. A stock price dataset from yesteryears is about as useful for today’s market insights as a cassette tape in the age of streaming. The value of data ages like milk, which applies across the board for any mission-critical decisions.
Attempting to wrangle a dataset with no clearly defined Usecases is akin to cart-pushing in the absence of a horse. Now, sprinkle in a pinch of hazy data governance, and you have a recipe for disaster. DQ’s struggles don’t just lead to restless nights and painful meetings; they strike at the very heart of Trust and Credibility, an irreversible and irreparable attack on the sacred sanctuary. Imagine an executive dashboard cobbled together from four different data sources. Its quality is no stronger than its weakest link. If one source isn’t playing by the data governance rulebook, it’s the chink in the armour that could bring the whole credibility crashing down, and all the glittering visualization will not earn back the lost faith in your reporting systems.
Like the enchanting symphony of spices in Biryani, Data Quality isn’t a lone obstacle but rather an intricate tapestry woven with threads of fuzzy use cases and governance gaps, too complex to savour. Attempting to whitewash all these challenges by procuring a shiny DQ tool from a fancy vendor is like trying to weather a hurricane with a paper umbrella. The real deal? The actual solution lies in forging a resilient data governance framework, meticulously addressing each detail and intricacy in the realm of analytics use cases. In the delicate dance of data, it’s not just doling out a set of Python transformations and ETL jobs; it’s about crafting a foundation of trust where every note of governance harmonizes with the melody of insights, ensuring that our data-driven journey resonates with unwavering integrity and purpose.
Shammy Narayanan is a Practice Head for Data and Analytics in a Healthcare organization, with 9x cloud certified he is deeply passionate about extracting value from the data and driving actionable insights. He can be reached at shammy45@gmail.com