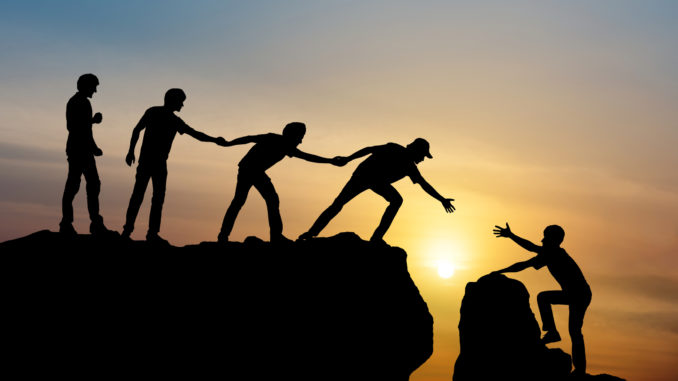
Think for a moment what it means for an organization to become truly data-driven. After committing to making decisions purely based on data, a company can drive out costly errors, gain efficiency, and embrace objectivity. Yet, it also effectively sidelines the human emotion and gut feelings that have traditionally guided business for centuries.More than just a gut feeling, however, is the concept of trust, which will rise in importance during this critical moment in the digital transformation of business. Those who extract insights from and make decisions with data must be able to trust that the data is accurate and complete.
For example, data scientists who have studied the famous “Moneyball” strategy applied in baseball place higher value on the actual decision from general manager Billy Beane to trust the data and change the organization so it could deliver on the data’s potential.Factoring in performance data to select players was not anything new; it was the commitment to the data that was revolutionary.The success rates of game-changing innovations like conversational commerce, AI, block chain, and IoT depend on this very crucial concept too. The most forward-thinking companies piloting new technology have committed to exploring revolutionary uses of data. However, they risk time and resources if the data feeding these experiments is not structured and collected consistently.
Most enterprise architects developing tomorrow’s platforms today have already realized that we can no longer operate in silos—it will simply kill any promise of agility. By prioritizing external collaboration and data sharing based on global data standards, multiple departments and functions can use a common language to lay the groundwork for enhancing trust in data and scaling technology effectively. Let’s take a look at how standards enhance trust in technology adoption, and form a much-needed bridge for companies making the leap from how they’ve always conducted business to new data-reliant strategies.
WHAT STANDARDS DO
Global data standards (such as the GS1 System of Standards) allow any company—from large multinationals to small startups—to identify their organizations,locations, and individual products accurately, authentically, and persistently. This is emerging as a fundamental prerequisite prior to adopting any new technology application. With standards helping companies connect the flow of data to the movement of products outside of their own proprietary systems, they are taking the crucial first step toward systems interoperability. Additionally, standards provide structure to the vast amount of data currently being collected for analytical purposes. As companies are evaluating the enrichment of the data they’re collecting, standards ensure that efficiency is always their top priority.
Historically, product identity consisted of the standard UPC or Global Trade Item Number (GTIN),along with any associated master data and logistics in-formation that drove efficiencies in B2B supply chain operations. But today, the consumer is craving a richer experience with products prior to purchasing them, and more information needs to be available for their consumption. Brand owners now need to provide details about each individual item, and place greater emphasis on its digital counterpart. Examples of this extended product attribution include information about product sourcing practices, a detailed listing of ingredients, and data related to the product’s life cycle and warranty.
Data is increasing exponentially, so it is becoming more difficult for companies to ensure accuracy and completeness in product attribution. In an eye-opening example, GS1 US, the information standards organization, recently worked with leaders of industry to measure accuracy and completeness between product labels vs. retailer websites. Only 54% of all product attributes were accurate, and not a single product was 100% ac-curate for all attributes contained in digital listings. It is essential for companies to focus on data quality now as a critical first step toward digital transformation.
FEEDING THE ALGORITHM
For data to be productive in business, it needs to be structured for consistency to move easily between disparate, automated systems. Take artificial intelligence (AI) and machine learning for example. Many companies are exploring machine learning including innovative ways to train AI to make use of the data they’ve collected. This could go beyond current predictive analytics practices,where teams can make inferences about what could hap-pen next based on data, to next-level prescriptive analytics, where the numbers actually tell them what to do about it. AI’s exception management capabilities give data the power to move in this way with little human intervention and represents a true commitment to data-driven strategies.
Given this critical dependence on structured data,there must be careful consideration given to data standardization and business process consistency to help AI function as intended. The foundational data required to make algorithms “smarter” ultimately rely on the common global format that standards provide.
One example of this is how eBay has leveraged AI to enhance its marketplace experience. The e-commerce giant uses its more than 20 years’ worth of data to power its personalization and recommendation algorithms, re-lying on a consistent data structure to curate the right products for the right user out of its 1.2 billion listings every day. eBay has been advocating for the improved identification of products online (specifically using UPCs) to create an important bridge between a product’s physical and digital identity.
Efforts like these have become mission critical to global trade, where entire supply chains are being modernized to connect buyers and sellers in real time. While there are numerous benefits for companies that make the leap to become data-led businesses, the humans responsible for making decisions based on data need to be confident that their systems are set up to produce re-liable and consistent results. By collaborating on standards now to structure data, it can be more effectively leveraged for successful solutions, creating a win-win scenario for both developers and users. A&G