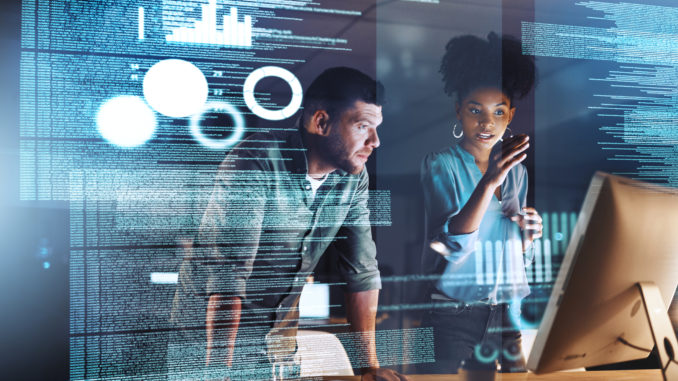
(Editor’s Note: This article is an approach paper for an organization’s analytics transformation journey. This article initially provides a brief overview of analytics and then provides a description about various types of analytics domains. At the end of the article is a table that depicts the roadmap of an organization for an analytics transformation journey.)
Analytics Overview
Analytics involves studying past historical data to research potential trends, to analyse the effects of events, or to evaluate the performance of a given tool or scenario. Analysing data help business managers make informed decisions to drive the company forward, improve efficiency, increase profits and achieve organisational goals. Analytics offers companies the ability to leverage data to impact their bottom lines. Data analytics, by definition refers to qualitative and quantitative techniques and processes used to enhance productivity and business gain. Data is extracted and categorized to identify and analyse patterns and behavioural data. By connecting data to action, organizations can understand consumer behaviour, anticipate future events, and gain new insights to build valuable relationship and Know the audience. The next paragraphs provide a brief description about various types analytics.
Business intelligence – BI: BI is the use of computing technologies for the identification, discovery and analysis of business data – like sales revenue, products, costs and incomes. BI technologies provide current, historical and predictive views of internally structured data for products and departments by establishing more effective decision-making and strategic operational insights through functions like online analytical processing (OLAP), reporting, predictive analytics, data/text mining, benchmarking and Business Performance Management – BPM.
Descriptive Analytics: As an analyst or a business owner, when you are looking for an answer of a question – What is happening in my business? This is when Descriptive analytics comes into place. It’s the most common and widely used analytics that analyses the data coming in real-time generally using effective visualisation tools like dashboards and allows us to learn from past behaviours and give us an idea about how they will impact future outcomes. But as it gives us only insight about whether everything is going well or not in our business, and it didn’t explain the root cause behind. This is the reason, highly data-driven businesses combine descriptive analytics with other types of data analytics to find the complete solution.
Diagnostic Analytics: When you already know what’s happening in your business using Descriptive analytics and you want to know that the answer of next question i.e. Why it is happening in your business or you want to know the root cause behind that, this is where Diagnostic analytics plays its part. Diagnostic analytics helps analysts or data scientists to drill down inside the data to find the answer. In business, BI dashboards help you drill down using hierarchies or do a quick comparison to find the reasons or factors that are affecting business.
Predictive Analytics: Predictive Analytics is based on what you get from descriptive and diagnostic analytics and used to find answers to the question of what is likely to happen in the future based on previous trends and patterns? This method is all about forecasting. Predictive Analytics utilizes various statistical and machine learning algorithms to provide recommendations and provide answers to questions related to what might happen in the future, that cannot be answered by BI. But as it’s probabilistic in nature, it just gives an estimate of a possible future outcome. Also, the accuracy is not 100% because it all depends on data quality, how you make educated guesses on the missing values and how and optimization is done.
Prescriptive Analytics: When you get the findings from Descriptive, Diagnostic and Predictive analytics like what’s happened, the root cause behind that and what-might-happen in future, Prescriptive model utilizes those answers to help you determine the best course of action to choose to bypass or eliminate future issues. You can use Prescriptive analytics to advise users on possible outcomes and what should they do to maximise their key business metrics. The best example in front of you is Google Maps which helps you to choose the best route considering distance, traffic and speed.
Machine Learning / Deep Learning: Machine learning is loosely interpreted to mean empowering computer systems with the ability to learn. The intention of ML is to enable machines to learn by themselves using the provided data and make accurate predictions. ML is a subset of artificial intelligence; in fact, it’s simply a technique for realizing AI. It is a method of training algorithms such that they can learn how to make decisions. Training in machine learning entails giving a lot of data to the algorithm and allowing it to learn more about the processed information.
Deep learning is a subset of ML: In fact, it’s simply a technique for realizing machine learning. In other words, DL is the next evolution of machine learning. DL algorithms are roughly inspired by the information processing patterns found in the human brain. Just like we use our brains to identify patterns and classify various types of information, deep learning algorithms can be taught to accomplish the same tasks for machines. The brain usually tries to decipher the information it receives. It achieves this through labelling and assigning the items into various categories. Whenever we receive a new information, the brain tries to compare it to a known item before making sense of it — which is the same concept deep learning algorithms employ.
AI/Cognitive Analytics: Cognitive analytics combines several intelligent technologies like artificial intelligence, machine-learning algorithms, deep learning etc.to apply human brain like intelligence to perform certain tasks. Basically, this type of analytics is inspired by how the human brain processes information, draws conclusions and codifies instincts and experience into learning such as understanding not only the words in a text but the full context of what is being written or spoken. All these intelligent technologies make a cognitive application smarter and more effective over time by learning from its interactions with data and with humans.
The views expressed in this article are the author’s views and AtoS does not subscribe to the substance, veracity or truthfulness of the said opinion.