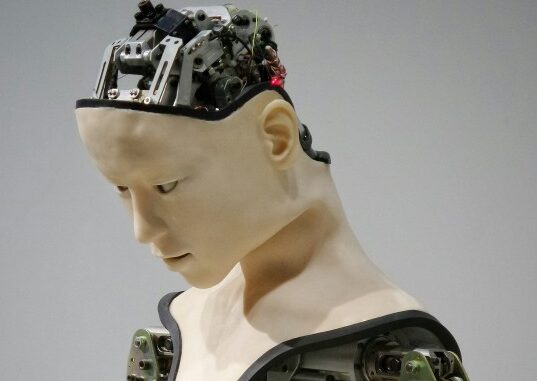
By Phil Robinson, Principal Security Consultant, Prism Infosec
When it comes to rolling out Generative AI (GenAI) in a business environment, the predominant concern is, will it put corporate data at risk? Is the large language model (LLM) able to ingest sensitive data and leak it to other users? Is there any danger of that learning leaking out into the wider world? And how do you lock it down without compromising results? It’s these issues that have been top of mind among those vendors releasing B2B versions of the technology.
These concerns are entirely justified and do need to be addressed. We saw Samsung, for example, temporarily ban the technology last year after its engineers inadvertently leaked confidential data by pasting source code into ChatGPT. In fact, one in five UK businesses have admitted to staff exposing company data via ChatGPT, according to a Riversafe survey conducted back in January. Granted these leaks were via a public LLM but proprietary versions may still be an issue.
If we look at Microsoft’s GenAI, Copilot, for instance, which is based on Microsoft Azure Open AI and has been widely integrated across its services and is in Windows 11, there have been multiple theoretical examples of how Copilot could be subverted. These include asking Copilot to return information on staff bonuses, files with credentials or details on M&A activity in what is being dubbed ‘prompt hacking’. These would stem from the technology not being configured correctly, however, and Microsoft is at pains to stress that user permissions must be assigned and least privilege enforced pre-deployment.
Other top risks
However, while data leakage is an issue it’s by no means the only one. GenAI stands apart due to its autonomous nature and its unique ability to create new content from the information it is exposed to, and this introduces a whole host of new problems.
Data poisoning, for instance, sees a malicious actor intentionally compromise the data feed of the AI to skew results. This might involve seeding an LLM with examples of deliberately vulnerable code resulting in issues being adopted in new code. Without proper checks and balances in place, this could result in the poisoned data being pulled into organisational codebases via requests from developers. The code could then end up in production application and services which would be vulnerable to a zero-day attack.
AI hallucinations, sometimes referred to as confabulations, are another issue. Unlike poisoning, this is the result of the AI’s autonomy which can see it make incorrect deductions based on the data its presented with. GenAI can and does make mistakes, and there are numerous notable examples here too. In a court case over a personal injury claim against Avianca airlines, the attorney used ChatGPT to look for legal precedents and ended up citing non-existent cases replete with false names, file numbers, citations and quotes. There’s also evidence to suggest that Amazon’s Q chatbot can hallucinate or return harmful or inappropriate responses, which brings us on to the topic of bias. This sees the AI not just misinterpret but begin to form distorted world views that are racist or misogynistic.
These misuses are all just as problematic as data leakage, yet a recent Infosecurity webinar poll found the vast majority (60%) of security professionals regard accidental leakage as the biggest threat when using AI tooling for security operations. Data poisoning and other attacks trailed at 26% and AI hallucinations at 13%. Interestingly, nobody regarded bias or discrimination against data sets as an issue even though this could prove hugely damaging.
Regulation and risk
However, if we look at the recommendations made by the Information Commissioner’s Office (ICO), which has sought to clarify data protection guidelines following the introduction of GenAI, all four feature highly. It stipulates that effective governance will require transparency and accuracy in processing, fairness and accountability, and the preservation of data integrity through data minimisation. But underpinning all of those are the age old security tenets of confidentiality, integrity and availability (CIA) which have never been more relevant.
But how can organisations now look to govern AI in a way that accommodates the nuances and peculiar exploits the technology is vulnerable to? Thankfully there are now a number of relevant frameworks that promised to make the task easier.
We saw the release of ISO/IEC 22989:2022 back in September which provides the necessary terminology and definitions needed to enable those tasked with governance to discuss it in a standard language. It provides the building blocks needed to create an AI taxonomy and governance framework against which controls can be assessed and measured. And it was followed in December by ISO/IEC 23053:2022 which sets out how AI can be provided and so is most likely to be used in commercial agreements and contracts between vendors and their corporate customers.
In February, ISO/IEC 23984:2023 was published which is much more focused on risk management. The standard identifies sources of risk associated with AI but it’s essentially based upon ISO 31000:2018 and uses the best practice risk management of the previous standard applied in an AI context. It’s meant to be used as a practical framework and so maps the risks of AI against possible processes and controls but it’s also flexible enough to lend itself to any organisation looking to govern the AI lifecycle.
The most well-known AI framework, however, is ISO/IEC 42001:2023. It focuses on the integration of AI Management Systems (AIMS) into existing processes within the organisation and is very comprehensive in addressing AI issues ranging from data privacy through to security and ethical considerations such as transparency. It addresses threats such as bias by advocating the use of different datasets and continuous monitoring so that instances of it occurring can be detected and arrested.
However, not all organisations will need to manage their own AI system. For those looking for a framework to govern risk in a variety of contexts, NIST’s AI Risk Management Framework (AI RMF) may well prove more appropriate. Released in January 2023, NIST has just issued the AI RMF Generative AI Profile this April which provides use cases to guide implementation. In it, NIST addresses the four risks – data leakage, poisoning, hallucinations and bias – among a total of twelve others. It then goes on to outline the controls that can be put in place to guard against these risks, setting them out under the pillars of Govern, Map, Measure and Manage, with a useful appendix on governance and pre-deployment considerations including practical steps such as red team testing.
In conclusion, AI governance is still much a learning curve for organisations but while it may be tempting to call a halt to deploying the technology, this risks the business losing out. Armed with these new governance frameworks, it is now possible to assess risk and devote the time necessary to carry out pre-deployment assessments and to configure these B2B LLMs correctly. Controls can then be put in place to catch any possible deviance in terms of the AI’s performance while achieving compliance will demonstrate the business has done its due diligence when it comes to safeguarding its data, people and processes.