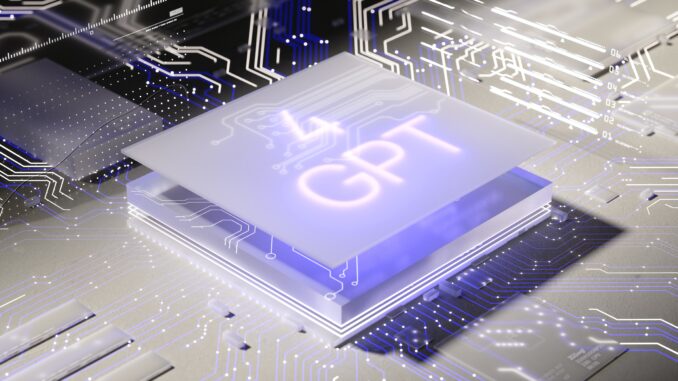
By Nate Sanders
GPT-3 does not have to be perfect to work.
That notion of perfection seems to be the sticking point utilized by critics of the current state of GPT-3 models, presenting the reliance on the technology as an all or nothing proposition.
Executives such as Bank of America CEO Brian Moynihan, who according to Bloomberg recently proclaimed that the lack of data-based responses with popular text generators make accuracy a challenge and thus not yet fit for corporate use, are misunderstanding the malleability of AI.
Sure, there are plenty of GPT-3 offerings and tools now, including the most ubiquitous large language model (LLM), Chat GPT, that have yet to ascend to the advanced levels required by many in enterprise architecture. But the ways in which current LLMs can be tailored to help infrastructure and operations are nearly limitless. AI tools can change a company’s entire approach to their systems, enhance decision making, optimize planning, and improve communication amongst stakeholders. These models can deliver accurate, dependable insights and predictions across every aspect of planning, security, and governance.
By fine-tuning LLMs to look for specific needles in giant haystacks of both unstructured and structured data, current GPT-3 models can monitor and enhance systems performance in a multitude of ways, such as:
Analyzing network logs
LLMs could be used to analyze logs generated by different systems and identify unusual behavior patterns. They can be a reasoner on complex logs, especially with systems that have not been sanitized or normalized by ETL. By analyzing the language used in the logs, a system could identify potential security breaches or areas for improvement in network security. It can find problems faster, make them easier to identify, and position teams to find solutions to the issues quicker and with better results.
By fine tuning the model on regular network patterns, or even better, giving it context around what irregular network patterns look like, a much more scalable first line of defense for network traffic can be created. By having a model that is not entirely driven by static rules and heuristics, but rather by the dynamics of language-based reasoning, it could reliably spot irregularities.
Input preprocessing— including schema mapping and normalization
If you have distributed datasets that you need collated by specific fields— for example an identity field, GPT-3 can be fine-tuned, and combined with a semantic search layer to assess the schema of the different tables, constraints metadata, and make sense of how each column could be mapped from the different datasets to a desired schema.
Governance and compliance monitoring: phishing attacks and fraud detection
LLMs can be used to analyze email messages and identify potential phishing attempts based on the language and content of the message. Without an engineer writing the heuristics, LLMs could analyze emails for spelling errors, differences in subject lines, poor grammar, etc.— anything that is white hot signal for phishing practices. They can then draft detailed insights and narratives, allowing them to attack the problem quickly.
AI models can also be used to analyze financial transactions and patterns of behavior, surfacing irregularities in real time, and identifying potentially fraudulent activity. Because LLMs are sequence-based models, the data could be fine-tuned to look for specific signals and irregularities. Even something as benign as spelling errors in VAT details could be better handled and automated with the help of LLMs reading through the data.
Across all aspects of systems and governance, it is possible to fine-tune LLM to give accurate and actionable results, increasing performance and optimizing resources.
Progress is better than perfection.
Nate is the co-founder of Artifact, artifact warehouses qualitative data at every critical stage of the customer journey, and then uses machine intelligence to build analyst-grade reports so you can find meaningful patterns and uncover customer needs.