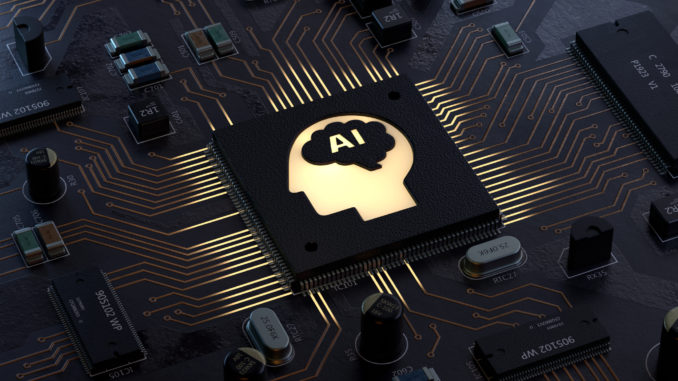
Over the years, as IT architecture professionals, we have been mastering our skills, capabilities, and experience when it comes to designing, building, operating, and having strong governance of any system as requested by organizations of all sizes. Technology has become an integral part of our daily life. It’s used everywhere at any time and has made it easier for many of us.
To make a system usable, we know how to design and implement it to meet run-time quality attributes such as performance, reliability, availability, and scalability. We know how to increase the system adoption and usage by using processes and tools (i.e., Prosci Methodology) to manage the people side of the equation to reach and empower every user before, during, and after the system’s launch. We know how to drive innovation and business growth using disruptive technologies such as Artificial Intelligence (AI), the Internet of Things (IoT), and Blockchain. Do we know how to integrate the ethics of AI into the design and development of our systems? Is our organization ready to implement a robust and what I call a Responsible AI governance process?
What is Responsible AI? Why is it so important?
To answer these questions, first, we need to ask ourselves what makes AI different from other technologies? Unlike any other technology, AI’s proximity to human intelligence and its power for both harm and good that is driving today’s conversation. Just think about it. We’re the first generation in humanity’s history to endow computers to make decisions that previously have always been made only by people. It’s therefore of fundamental importance that we get this right.; that we imbue computers with the capacity to think ethically and to aspire to the best of what humanity has to offer.
Despite all its benefits, AI could unintentionally mistreat people or reinforce existing societal biases. Over the past couple of years, we’ve seen many news stories about AI systems that are used to allocate or withhold opportunities, resources, or information in domains like criminal justice employment and hiring, finance credit. For example, imagine a financial lending institution had developed a risk scoring system for their loan approvals using training data indicating that loan officers have historically favored male borrowers. Considering this, the model may approve most of the loans to male borrowers. Without an audit, this unfairness would’ve persisted in the system, unintentionally adversely affecting most of the institution’s users.
On August 18, 2020, Gartner added Responsible AI as new category in its Hyper Cycle for Emerging Technologies. It defines Responsible AI as a series of technologies enabling use cases that involve improving business and societal value, reducing risk, increasing trust and transparency, and reducing bias mitigation with AI.
From my point of view, Responsible AI is about how do we design, develop, and deploy systems based on AI technology to make sure they are fair, reliable, safe, and trustworthy. We need to go beyond just improving the data and models; we also must think about the people who will ultimately be interacting with our AI systems.
Sensitive categories of AI systems
Sensitive uses involve AI-powered automated decisions or recommendations that can have a broad impact on people’s lives. A development or deployment scenario is considered a sensitive use if it falls into one or more of the following categories:
- Denial of consequential services. The scenario involves using AI in a way that may directly result in the denial of consequential services or support to an individual. For example, imagine that an AI system can generate insights about an employee’s performance at work, which is used as part of a performance management process that could affect employee compensation or promotion.
- Risk of harm. The scenario involves using AI in a way that may create a significant risk of physical or emotional harm to an individual. An example of this category could be an AI system is used to control machinery in a factory where people work and interact with that machinery. Another example is an AI system in charge of locking or unlocking emergency doors based on the data provided by the sensors available in a building.
- Infringement of human rights. The scenario involves the use of AI in a way that may infringe on human rights. Human rights that can be implicated by AI systems include the right to privacy, freedom of expression, peaceful assembly and association, freedom from discrimination, and the right to life, liberty, and security. For example, imagine an AI system deployed by law enforcement to conduct ongoing surveillance of individuals at public protests, which could interfere with the right to freedom of assembly, association, and expression.
We should look to identify sensitive uses as early as possible in the sales, design, and definition process. In case of a potential case, we should pause to better understand the risks, and report it to seek for guidance.
Sources of AI risk
By now, you would logically conclude that AI can create possible harm around fairness, reliability, safety, and privacy. These not only pose a risk to the organization but end-users and society at large. These risks often result from issues with the data, the AI model itself, or the model’s usage scenario.
- Data: Before machine learning models are used, they are trained to recognize patterns using “training data.” Organizations need to be careful and considerate about what training data is used and how it is structured. Flawed training data will create flawed AI models. If there’s only data from one age group or one time of year, for example, the AI model will end up skewed or biased. Risks can arise if the data has errors, lacks critical variables or historical depth, is an insufficient sample size, or doesn’t match the model’s deployment context.
- Model: AI models can also create risks if they are not well-designed, which could cause them to make incorrect approximations, choose an inappropriate objective to optimize, or use a variable as a proxy for another in a way that unintentionally introduces bias. Organizations need to consider potential issues before building their model. After it’s built, they can monitor model performance and accuracy during the training process and on an ongoing basis, and re-train the model periodically. AI models can “drift” or decline in performance over time. Even if a model performs well, it should be updated regularly to take advantage of more recent training data.
- Usage scenario: Each usage scenario may contain potential harms and be subjected to risk assessment and governance approval. It’s essential to ensure that each AI model is only used for the purpose for which it was designed and approved.
With such inherent risks, organizations must maintain oversight and control of AI solutions to ensure compliance with regulations and ethical principles.
Transparency in AI
When designing AI systems, we must ensure that the purpose and the level of transparency needed are well-defined and documented.
We should start by conducting an assessment based on their users’ potential impact, interdependency with other IT systems, and regulatory obligations. Based on the results, we should build the following three components of transparency into our AI system:
- Traceability: Document goals, definitions, design choices, and any assumptions during the development process. We also need to document the provenance, source, and quality of initial training datasets and additional datasets for re-training.
- Communication: Be forthcoming about when, why, and how we choose to build and deploy AI, as well as the system’s limitations.
- Intelligibility – This refers to people’s ability to understand and monitor the technical behavior of an AI system. But merely publishing the underlying algorithms and datasets rarely provides meaningful transparency, as these can be mostly incomprehensible to most people, particularly with more complex systems like deep neural networks. Luckily, several promising approaches to achieving intelligibility are emerging. Some facilitate understanding of key characteristics of the datasets used to train and test models. Others focus on explaining why individual outputs were produced, or predictions were made. Even more, offer simplified but human-understandable explanations for a trained model’s overall behavior or the entire AI system. My advice is to explore the range of available intelligibility approaches and select those that most effectively provide the information about the system or its components that each stakeholder needs to understand to meet their goals.
AI Principles
Any organization should have a core set of principles that architects, developers, and any IT professional must follow for the responsible development and deployment of AI technologies.
As an example, at Microsoft we have six principles to guide our work around AI.
AI systems should treat all people fairly, be inclusive of empowering everyone, performing reliably and safely, being understandable, and respect privacy and be secure. Ultimately, people should supplement AI decisions with sound human judgment and be held accountable for consequential decisions affecting others and how their systems operate.
Of course, having a principle doesn’t always tell us how a difficult ethical question should be resolved. But at least we know what we’re aiming for.
With over 20 years of experience in IT industry, Pablo Junco is the Chief Technology Officer (CTO) for Microsoft Latin America & the Caribbean. He is passionate about exploring how technologies such as Open API, IoT, AI, and Blockchain can be used to change people’s lives. Pablo is currently managing a team of account aligned architects who are collectively accountable for driving digital transformation, innovation, and technical strategy to our customers.
Pablo is an IASA Certified IT Architect – Professional (CITA-P) and holds a Bachelor of Science from the University of Southern Mississippi (Institution of Spain).
PABLO JUNCO ON SOCIAL MEDIA
- LinkedIn: https://www.linkedin.com/in/pablojunco
- Twitter: https://twitter.com/pjuncob @pjuncob