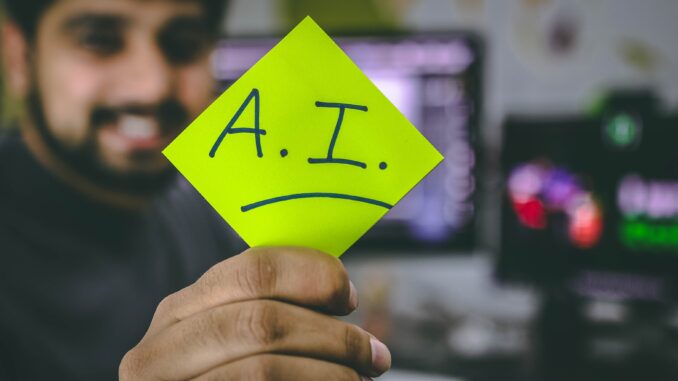
By Walson Lee
Abstract
This article presents a set of recommendations for enterprises planning to utilize generative AI. It introduces the concept of “Knowledge Engineering,” a modern take on the traditional practice of knowledge management, and highlights its importance in preparing for the use of generative AI. The article argues that combining Knowledge Engineering with Prompt Engineering can help enterprises effectively utilize their data and proprietary knowledge, offering a comprehensive strategy to navigate the generative AI landscape and maximize return on investment.
Introduction – Importance of Generative AI for Enterprises
Generative AI is indeed a once-in-a-generation technology game-changer for enterprises. Rapid advancements in various Large Language Models (LLMs) and significant investments by leading technology companies have made Generative AI a focal point for enterprises.
Many enterprises have already begun experimenting with Generative AI, with some implementing it in production. There are numerous examples of how this technology can improve all aspects of operations, including employee productivity, customer satisfaction and retention, and operational efficiency.
With proper design and implementation, a Generative AI-based business solution can offer potential cost savings and scalability. For instance, large healthcare organizations with multiple hospitals can use such solutions to streamline doctors’ and nurses’ daily routines, allowing them more time to focus on primary clinical tasks.
In this era of digital transformation, staying abreast of technological advancements is crucial for maintaining a competitive edge.
Prompt Engineering & Retrieval-Augmented Generation (RAG)
Generative AI-based solutions in an enterprise must meet several key requirements:
- Accuracy: The content generated by AI must achieve an acceptable accuracy rate depending on use cases and targeted user base.
- Reliability: Solutions should reference reliable source materials from operational systems or databases.
- Contextual Relevance: Utilize institutional knowledge or IP to provide contextually relevant content.
- Scalability: Ensure that solutions are scalable & cost-effective.
Two widely adopted techniques in Generative AI to meet these requirements are Prompt Engineering & Retrieval-Augmented Generation (RAG):
Prompt Engineering helps models better comprehend & respond to queries by crafting effective prompts optimizing quality and relevance. It determines output quality & relevance; effective prompts guide models to generate desired outputs while avoiding undesirable ones.
Grounding in Generative AI refers to the process of connecting abstract knowledge in AI systems to tangible, real-world instances. This is crucial for enterprise AI solutions to effectively address the outlined requirements. Grounding enhances an AI’s predictive and responsive capabilities by utilizing specific, contextually relevant information. In Generative AI, grounding involves equipping large language models (LLMs) with access to use-case specific data not included in their original training sets. The goal is to develop AI solutions that are adept at operating intelligently and effectively in real-world scenarios, delivering contextually appropriate and accurate results.
Retrieval-Augmented Generation (RAG) optimizes LLM output by referencing an external authoritative knowledge base before generating responses. This extends LLMs’ capabilities to specific domains or organizational internal knowledge bases without retraining them, offering a cost-effective solution for enhancing their relevance and accuracy across various contexts.
Knowledge Engineering in an Enterprise Environment
Knowledge Engineering is essential for implementing the RAG pattern effectively within enterprises. It encompasses the acquisition, identification, and classification of diverse knowledge types within an organization.
Acquisition of Knowledge
This initial step involves collecting pertinent data from various enterprise sources including databases, documents, reports, and employees’ tacit knowledge. Given the challenges posed by data volume, variety of formats, and sensitivity issues; enterprises can leverage data mining techniques and natural language processing tools for efficient extraction and structuring of information while adhering to stringent data governance policies ensuring security and privacy.
Identification of Knowledge
This phase entails recognizing different types of knowledge and assessing their relevance. Challenges such as ambiguous or incomplete information can be addressed using machine learning algorithms that identify patterns and relationships in data; thus, classifying it effectively.
Classification of Knowledge
Herein lies the task of organizing identified knowledge into structured categories or taxonomies for easy accessibility by AI models. Overcoming challenges related to creating a comprehensive yet scalable classification system can be achieved through ontology modeling techniques coupled with collaboration between domain experts and Data Scientists/AI Engineers for effective categorization.
In essence, overcoming these challenges ensures that AIs are well-grounded in relevant structured information enabling them to provide effective generative AI applications.
Knowledge Maturity Model: A Human Brain-Inspired Approach
The “Knowledge Maturity Model” is a novel approach to Knowledge Engineering that mirrors the functioning of the human brain’s memory system. It categorizes knowledge into three distinct types: short-term, intermediate-term, and long-term, each possessing unique characteristics and applications.
- Short-term knowledge is transient and frequently updated. It encompasses insights from recent customer activities or financial transactions. Enterprises leverage this type of knowledge to identify emerging trends or respond swiftly to customer feedback.
- Intermediate-term knowledge accumulates over a period (e.g., 3 months to 2 years) and is often associated with specific business groups or processes. Examples include operational procedures for mitigating COVID-19 risks or financial trending models. This type of knowledge guides strategic decision-making and helps enterprises adapt to evolving circumstances.
- Long-term knowledge constitutes the enterprise’s institutional wisdom or intellectual property amassed over extended periods. It encompasses company policies and foundational business principles that rarely change, offering a stable framework for operations.
Implementing the Knowledge Maturity Model entails identifying and classifying an enterprise’s existing knowledge into these categories—a process streamlined by AI technologies capable of analyzing vast data volumes to extract pertinent information. Once classified, this knowledge can be effectively employed alongside Generative AI technologies.
Enterprises stand to gain manifold benefits from this model—it not only enhances Knowledge Engineering efficacy but also ensures valuable insights are retained and readily accessible when needed. By aligning with human memory’s natural functioning, it fosters more intuitive and efficient AI systems—making it a promising approach for those keen on maximizing Generative AI’s potential.
Using Prompt Engineering with the Knowledge Maturity Model
Successful implementation hinges on precise identification and classification of each source of enterprise knowledge—a collaborative effort involving AI specialists like Prompt Engineers, Data Analysts, and in-house domain experts.
The initial step involves identifying various enterprise-wide sources of information before classifying them according to their nature (short-term, intermediate-term, or long-term) based on their volatility.
Maintenance and timely updates are crucial for short-term/intermediate term knowledges’ relevance—ensuring that they remain current enhances Generative AI effectiveness significantly.
Conclusion
In conclusion, we have proposed a Knowledge Engineering model and highlighted the importance of combining Prompt and Knowledge Engineering. This dual approach enables enterprises to effectively harness the power of Generative AI, a once-in-a-generation game changer.
Given the rapid advancements in AI technology, it is imperative for enterprises to start putting action plans in place today. The urgency to adopt these practices cannot be overstated. However, enterprises must also be aware of the potential risks or challenges that they might face during this transition.
These challenges could include data privacy issues, the need for significant investment in AI infrastructure, and the requirement for ongoing training and development for staff. However, with careful planning and strategic investment, these challenges can be mitigated.
By embracing the dual power of Knowledge and Prompt Engineering, enterprises can navigate the Generative AI landscape effectively and maximize their return on investment. The future of enterprise operations lies in the successful integration of these advanced technologies.